A Deep Learning System for the Automated Calliper Placement to Measure Multiple Fetal Brain Structures from Two-Dimensional Ultrasound Images
Objective
To evaluate the performance of a deep learning (DL) system for automated calliper placement to obtain 6 key sonographic measurements of the fetal brain (transventricular [TV] and transcerebellar [TC] planes).
Methods
From 3 centres (2 tertiary referral centres, 1 routine imaging centre), 1497 (583 pregnancies) TV, and 596 (187 pregnancies) TC plane images were obtained retrospectively using 3 commercial ultrasound devices (GE Voluson E8, S10, P8). The calliper positions (X and Y coordinates) for 6 measurements (TV plane: biparietal diameter [BPD], occipitofrontal diameter [OFD], atrial width [AW]; TC plane: transcerebellar diameter [TCD], cisterna magna size [CMS], nuchal fold thickness [NFT]) provided by fetal medicine specialists (FMS) were used as the gold standard. For each measurement, we trained (1200 images/measurement) a DL system (high-resolution network [HR-Net]) to automatically predict the calliper positions (2 per measurement) using the gold standard dataset, and measurements were computed as the Euclidean distance between them. We assessed the performance (calliper position, measurement) of the DL system (vs. 2 FMS) on an independent (unseen) test set of 145 images (145 pregnancies) by computing the mean Euclidean error (DL system vs. 2 FMS) and the absolute agreement (intraclass correlation coefficients [ICC]; two-way random-effects, average rater) for each measurement.
Results
For all 6 measurements, the Euclidean errors (means) were always less than 2.11±0.98mm, and the DL system was in a good (NFT, CMS; ICC > 0.80) to excellent (BPD, OFD, TCD, AW; ICC > 0.90) agreement with 2 FMS.
Conclusion
The successful clinical translation of the proposed DL system is of high value for training novice users and in low-resource settings that lack well-trained specialists for obtaining reliable fetal structural measurements.

The dotted red lines in each graph represent the 95% Confidence Interval (CI)
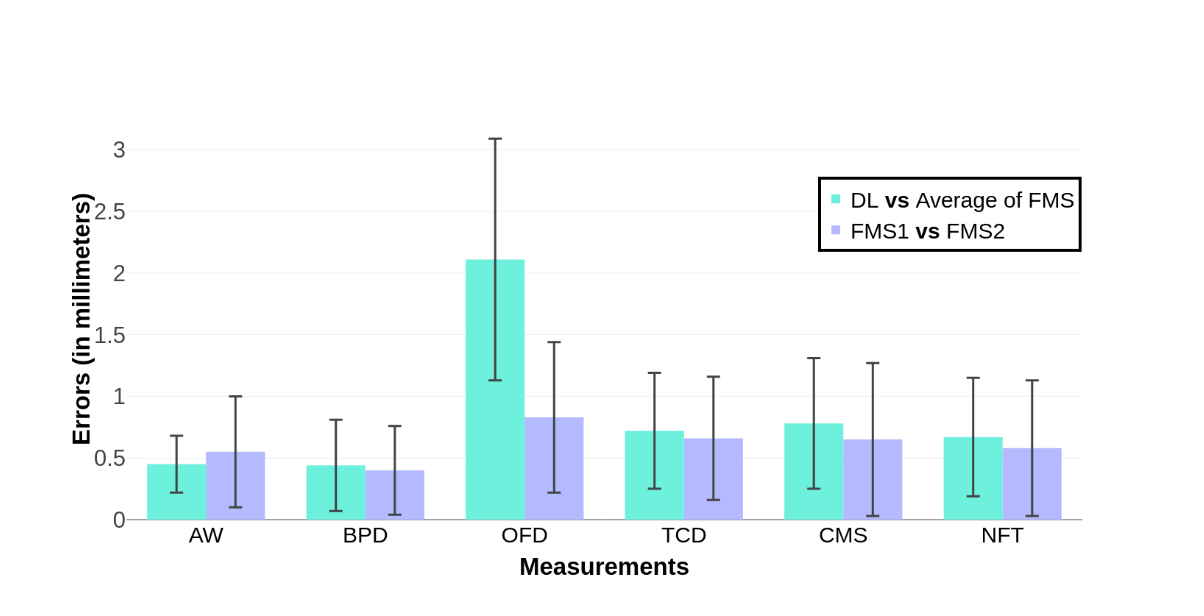